Systems biology goes public
15 Feb 2018 by Evoluted New Media
A personalised approach to medicine based on systems biology can only happen if there is a huge change in the way we democratize data. Data silos have to be a thing of the past if we are to develop the models needed, and leveraging publicly available databases will be vital
Precision medicine is predicated on the comprehensive understanding of the patient. Yet in a world of over seven billion unique individuals, how can scientists tailor a treatment based upon the genome, metabolome, or even microbiome?
In today’s biopharmaceutical industry, which faces rising costs and lower R&D productivity, it often seems more prudent to disregard the smaller variances that make each of us unique and target larger patient populations in an attempt to capture more value. However, pressure from consumers and regulatory bodies is placing a premium on demonstrable benefits for each new therapeutic, which can be difficult to show in a homogenised body of heterogeneous patients. As a result, scientists are looking to new sources of data, tools, and methodologies to improve patient outcomes with bespoke treatments.
The modelling and simulation of biological processes has become a staple in biopharmaceutical R&D, and for good reason. Over forty years of research have gone into the study of in silico chemical reaction modelling, with the first model of the action of a protein, bovine pancreatic trypsin inhibitor, published in 19771. This work would eventually contribute to the 2013 Nobel Prize in Chemistry as well as a vibrant community of protein modellers worldwide2. One of the key benefits that biological modelling and simulation offers is the ability to leverage existing data when preparing new hypotheses, effectively helping scientists decide what to test next.
It is important to note that in silico experimentation and physical experimentation are not at odds with each other; rather, they create a feedback loop to support decision-making. A model can use existing data to make a prediction, which can be verified at the lab bench, which can in turn help refine the existing model for improved predictions or create a new model. For example, to look at a small variation in protein structure such as swapping a leucine for an isoleucine, a team of scientists would first need to create a plasmid and cell line to synthesise the mutant protein, synthesise any ligands needed for the assay, and run the assay to determine any changes in activity. Running a simulation of this activity, however, could shorten the weeks of work needed at the bench to a couple of hours. This allows scientists to “pre-screen” hypotheses in a low-risk, low-resource environment, pushing attrition rates farther upstream in discovery and allowing teams to focus on only the highest quality candidates. This would also help make precision medicine-focused studies more economically feasible, as scientists could more easily study the effects of minute variations in DNA among different patient populations.
A complex web While modelling and simulation has helped to transform biopharmaceuticals, it is not a silver bullet. Until recently, the majority of modelling and simulation focused primarily on drug-protein or protein-protein interactions. This has limited the scope of the applicability of modelling and simulation as many of the most malignant diseases today, such as Alzheimer’s or cancer, are exceedingly complex and manifest via multiple mechanisms of action. These often require the study of large webs of protein signalling cascades rather than a single interaction. This limited scope also makes it difficult to incorporate some other types of variation among patient populations such as metabolomics data. As a result, the concept of biological modelling and simulation must be expanded to a more systemic level to take on these medical challenges. Enter the relatively new field of systems biology.
The goal of systems biology is “understanding how a biological system functions” – with ‘understanding’ defined operationally as the ability to describe the system on the basis of the characteristics of its components3. Adopting this more holistic approach allows scientists to blend together a wide variety of data types to enhance their decision making. This translates to a deeper understanding of how individual drug-protein interactions translate to changes on a cellular level. Additionally, elucidating existing mechanisms of these drug-protein interactions – both for therapeutic efficacy and safety – can allow scientists to more accurately predict outcomes in novel mechanisms, shortening the time it takes for related or multidrug therapies to be developed4.
Coupling these systemic models with traditional drug-protein or protein-protein models creates a sort of “multiscale” effect. As scientists begin to develop a better understanding of how a potential therapeutic affects a protein system, they can begin to predict the effects of related therapeutics on the same system in silico. “Zooming” in or out can allow for scientists to more finely tune the desired effects of their therapeutics based on variations between individual patient populations, supporting the drive towards true precision medicine.
Computational nirvana While the concept of being able to quickly model the effects of a novel therapeutic upon biological systems from the protein to cellular or tissue level sounds like a computational biologist’s nirvana, there are significant hurdles that stand in the way. The key challenge in systems biology is determining the model’s complexity, which is highly dependent on the amount of information available and the type of question that is being asked. In some instances, a qualitative “yes or no” may be sufficient to inform a decision (such as in whether or not inhibiting Protein A affects the downstream effects of Protein B). However, the more mechanistic models needed for precision medicine require more detailed information (such as how much will changing leucine 241 to an isoleucine in Protein A diminish Protein B’s downstream effects). For an individual research group, gathering the data required to answer some of these more complex questions, especially those pertaining to individual variations within a given patient population, may be prohibitively difficult and time consuming.
To achieve a culture of systemic modelling supplemented by publicly available data, research teams will need to overcome a major roadblock: siloed dataFortunately today’s scientific research does not happen in a bubble; thousands of individual scientists and groups of researchers are working worldwide, generating a rapidly growing global body of knowledge. This provides a unique opportunity for a pharmaceutical industry hungry for new sources of innovation – especially as more organisations decentralise and adopt external research partners. Much of this information is now being captured in publicly available databases such as UniProt, Reactome, and hundreds of others. These ever-growing bodies of data can be leveraged to develop the more complex and mechanistic systems models scientists require for better-tailored therapies, helping to fill any gaps in their own data and better support their decisions.
Previously, these gaps in a model would need to be filled by cycles of physical experiments on a case-by-case basis, lengthening the time it took to pass from conception to the bedside. By supplementing this systems-backed approach with publicly available data, scientists can shorten the time to discovery and fail more low-quality candidates while using up fewer resources. Additionally, advanced users can begin to blend together sets of previously unrelated data to identify trends within individual patient populations, improving outcomes with more precise medicine.
Road block To achieve a culture of multidisciplinary, multiscale systemic modelling supplemented by publicly available data, research teams will need to overcome a major roadblock in today’s public database ecosystem: siloed data. Today there are hundreds of different databases which may use different data standards and require extensive manual work to collate. This not only takes more time; manual methods are also prone to error, compromising the quality of the analysis. Data pipelining tools can help in this regard, as scientists can begin to automate some of the data blending for their analyses and mitigate some of the risks of manual data management.
Scientists can also share their workflows with less experienced colleagues to ensure consistent analysis standards within an organisation. However, this drive towards widespread in silico modelling will need a concerted effort across the industry and academia at large to curate these databases, build bridges between them, and democratise their benefits.
One-size-fits-all treatments are becoming a thing of the past as scientists begin to leverage the sea of data at their fingertips. Public databases are beginning to transition from reference tools to actionable data sets that can augment research. Additionally, as life science modelling and simulation continues to mature, deriving the effects of therapeutics on their target protein pathways from the individual protein to the systemic level, the ability to access a wider body of data can help scientists develop more mature and targeted models faster and more efficiently than ever before.
While building bridges among hundreds of public databases remains a significant challenge, systems biology can save significant time and resources in driving successful biopharma projects forward.
References
- McCammon JA, Gelin BR, Karplus M. (1977). Dynamics of folded proteins. Nature, 267, pp. 585–590.
- https://www.nobelprize.org/nobel_prizes/chemistry/laureates/2013/karplus-bio.html [Accessed 31/8/2017]
- Snoep JL, Westerhoff HV. (2005). From isolation to integration, a systems biology approach for building the Silicon Cell. In: Alberghina L, Westerhoff HV., ed., Systems Biology: Definitions and Perspectives, 13th Berlin: Springer AG, pp. 13-30.
- Visser SAG, de Alwis DP, Kerbusch T, Stone JA, Allerheiligen SRB. (2014). Implementation of Quantitative and Systems Pharmacology in Large Pharma. CPT Pharmacometrics Syst Pharmacol, 3(142). Available at: https://www.ncbi.nlm.nih.gov/pubmed/25338195 [Accessed 31/8/2017].
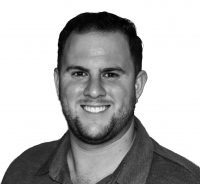